Biometrics Fingerprint Recognition Pdf Writer
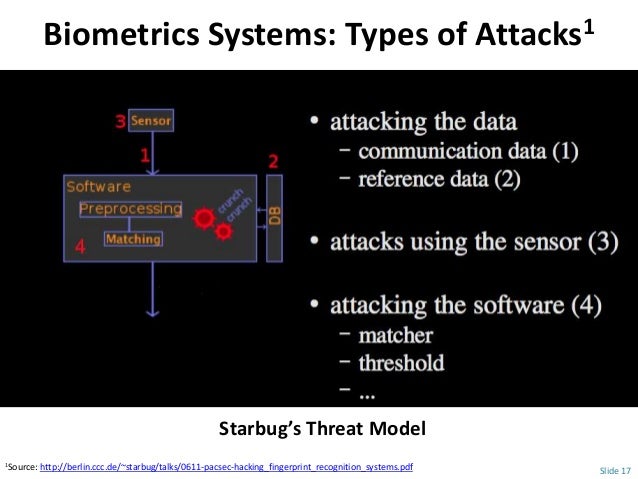
Commonly implemented or studied biometric modalities include fingerprint, face, iris, voice, hand writer recognition, and hand geometry. There is not one biometric modality that is best for all implementations, and many factors must be taken into account when implementing a biometric device. More information (pdf). Futronic's FS26 USB2.0 Fingerprint Mifare Card Reader/Writer combines a fingerprint scanner and an. The use of fingerprint recognition for logical and physical.
Whether dealing with employee contracts, sensitive corporate documents, presentations or other documents, scanning has become a vital business tool. There are several different scanning programs and services, ranging from basic software to document services that protect your scanned data through encryption and 24-hour surveillance.
Regardless of which system you adopt, having a scanning tool will allow your business to thrive, as more businesses move toward paperless operation. Scanning also assists with organization and productivity. By scanning company documents, important information is uploaded and stored in a cloud-based system that is searchable.
This makes files accessible and easy to find, as opposed to having to dig through hundreds of paper files in search of one document. OmniPage's simple interface and easy-to-install software make it our top pick for best scanning software for PC. OmniPage uses 3D correction technology that deskews images and improves scanning accuracy. The program automatically detects languages and spell checks the document, setting it apart from competitors as a great foreign language scanner. If your business manages international documents in different languages, OmniPage is a great option.
It can even handle a Latin-based alphabet as well as traditional and simplified Japanese, Korean and Chinese characters. Document scanning is an expanding business practice. Businesses rely on it when sending signed documents or other paperless forms to employees or clients in remote locations. Insurance, healthcare and legal businesses, in particular, rely on document scanning and cloud-based storage. According to a survey posted on, 75 percent of respondents were looking to adopt some form of a cloud content management system. Other suggests that cloud-based document scanning is being used to expedite workflows and add flexibility to document storage. As more businesses turn to document scanning services to streamline processes and add more storage options, security has become an important aspect of document scanning.
ISACA, an IT governance association, 53 percent of enterprises experienced more attacks in 2017 compared to 2016. Of the attacks, 50 percent were motivated by financial gain while 37 percent were motivated by stealing personal information.
Only 53 percent of businesses said they had a formal response process to deal with ransomware attacks. For businesses looking to transition sensitive records to a fully digital platform, cybersecurity is a major consideration. Some document scanning companies go to great lengths to ensure that your business's files are safe from the threat of cyberattack.
1 Introduction and Fundamental Concepts From a very young age, most humans recognize each other easily. A familiar voice, face, or manner of moving helps to identify members of the family—a mother, father, or other caregiver—and can give us comfort, comradeship, and safety. When we find ourselves among strangers, when we fail to recognize the individuals around us, we are more prone to caution and concern about our safety. This human faculty of recognizing others is not foolproof. We can be misled by similarities in appearance or manners of dress—a mimic may convince us we are listening to a well-known celebrity, and casual acquaintances may be incapable of detecting differences between identical twins.
Nonetheless, although this mechanism can sometimes lead to error, it remains a way for members of small communities to identify one another. As we seek to recognize individuals as members of larger communities, however, or to recognize them at a scale and speed that could dull our perceptions, we need to find ways to automate such recognition. Biometrics is the automated recognition of individuals based on their behavioral and biological characteristics. 1 “Biometrics” today carries two meanings, both in wide use. (See and.) The subject of the current report—the automatic recognition of individuals based on biological and behavioral traits—is one meaning, apparently dating from the early 1980s. However, in biology, agriculture, medicine, public health, demography, actuarial science, and fields related to these, biometrics, biometry, and biostatistics refer almost synonymously to statistical and mathematical methods for analyzing data in the biological sciences. The two usages of.
And fingerprinting were first deployed commercially in the 1970s, almost immediately leading to concerns over spoofing and privacy. Larger pilot projects for banking and government applications became popular in the 1980s.
By the 1990s, the fully automated systems for both government and commercial applications used many different technologies, including iris and face recognition. Clearly both meanings of biometrics are well-established and appropriate and will persist for some time.
However, in distinguishing our topic from biometrics in its biostatistical sense, one must note the curiosity that two fields so linked in Galton’s work should a century later have few points of contact. Galton wished to reveal the human manifestations of his cousin Charles Darwin’s theories by classifying and quantifying personal characteristics. He collected 8,000 fingerprint sets, published three books on fingerprinting in four years, and proposed the Galton fingerprint classification system extended in India by Azizul Haque for Edward Henry, Inspector General of Police, in Bengal. It was documented in Henry’s book Classification and Uses of Finger Prints. Scotland Yard adopted this classification scheme in 1901 and still uses it. But not all of Galton’s legacy is positive.
He believed that physical appearances could indicate criminal propensity and coined the term “eugenics,” which was later used to horrific ends by the Third Reich. Many note that governments have not always used biologically derived data on humans for positive ends. Galton’s work was for understanding biological data. And yet biostatisticians, who have addressed many challenges in the fast-moving biosciences, have been little involved in biometric recognition research. And while very sophisticated statistical methods are used for the signal analysis and pattern recognition aspects of biometric technology, the systems and population sampling issues that affect performance in practice may not be fully appreciated. That fields once related are now separate may reflect that biometric recognition is scientifically less basic than other areas of interest, or that funding for open research is lacking, or even that most universities have no ongoing research in biometric recognition.
A historical separation between scientifically based empirical methods developed specifically in a forensic context and similar methods more widely vetted in the open scientific community has been noted in other contexts and may also play a role here. Galton, Fingerprints (1892); Decipherment of Blurred Finger Prints (1893); and Fingerprint Directories (1895). All were published by Macmillan in London. 3 National Research Council, The Polygraph and Lie Detection (2003).
Washington, D.C.: The National Academies Press, and National Research Council, Strengthening Forensic Science in the United States: A Path Forward (2009), Washington, D.C.: The National Academies Press. 4 For more on the history of the field and related topics, see F. Galton, On Personal Description, Dublin, Ireland: Medical Press and Circular (1888), and S.J. Gould, The Mis-measure of Man, New York: Norton (1981). BOX 1.2 A Further Note on the Definition of Biometrics The committee defines biometrics as the automated recognition of individuals based on their behavioral and biological characteristics.
This definition is consistent with that adopted by the U.S. Government’s Biometric Consortium in 1995. “Recognition” does not connote absolute certainty. The biometric systems that the committee considers always recognize with some level of error. This report is concerned only with the recognition of human individuals, although the above definition could include automated systems for the recognition of animals. The definition used here avoids the perennial philosophical debate over the differences between “persons” and “bodies.” For human biometrics, an individual can only be a “body”.
In essence, when applied to humans, biometric systems are automated methods for recognizing bodies using their biological and behavioral characteristics. The word “individual” in the definition also limits biometrics to recognizing single bodies, not group characteristics (either normal or pathological). Biometrics as defined in this report is therefore not the tool of a demographer or a medical diagnostician nor is biometrics as defined here applicable to deception detection or analysis of human intent. The use of the conjunction “and” in the phrase “biological and behavioral characteristics” acknowledges that biometrics is about recognizing individuals from observations that draw on biology and behaviors. The characteristics observable by a sensing apparatus will depend on current and, to the extent that the body records them, previous activities (for example, scars, illness aftereffects, physical symptoms of drug use, and so on).
Martin and J. Barresi, Personal Identity, Malden, Mass.: Blackwell Publishing (2003); L.R. Baker, Persons and Bodies: A Constitution View, Cambridge, England: Cambridge University Press (2000). Many traits that lend themselves to automated recognition have been studied, including the face, voice, fingerprint, and iris.
A key characteristic of our definition of biometrics is the use of “automatic,” which implies, at least here, that digital computers have been used. Computers, in turn, require instructions for executing pattern recognition algorithms on trait samples received from sensors. Because biometric systems use sensed traits to recognize individuals, privacy, legal, and sociological factors are. Involved in all applications.
Biometrics in this sense sits at the intersection of biological, behavioral, social, legal, statistical, mathematical, and computer sciences as well as sensor physics and philosophy. It is no wonder that this complex set of technologies called biometrics has fascinated the government and the public for decades. The FBI’s Integrated Automatic Fingerprint Identification System (IAFIS) and smaller local, state, and regional criminal fingerprinting systems have been a tremendous success, leading to the arrest and conviction of thousands of criminals and keeping known criminals from positions of trust in, say, teaching. Biometrics-based access control systems have been in continuous, successful use for three decades at the University of Georgia and have been used tens of thousands of times daily for more than 10 years at San Francisco International Airport and Walt Disney World. There are challenges, however.
For nearly 50 years, the promise of biometrics has outpaced the application of the technology. Many have been attracted to the field, only to leave as companies go bankrupt.
In 1981, a writer in the New York Times noted that “while long on ideas, the business has been short on profits.” The statement continues to be true nearly three decades later. Technology advances promised that biometrics could solve a plethora of problems, including the enhancement of security, and led to growth in availability of commercial biometric systems. While some of these systems can be effective for the problem they are designed to solve, they often have unforeseen operational limitations. Government attempts to apply biometrics to border crossing, driver licenses, and social services have met with both success and failure. The reason for failure and the limitations of systems are varied and mostly ill understood. Indeed, systematic examinations that provide lessons learned from failed systems would undoubtedly be of value, but such an undertaking was beyond the scope of this report. Even a cursory look at such systems shows that multiple factors affect whether a biometric system achieves its goals.
The next section, on the systems perspective, makes this point. THE SYSTEMS PERSPECTIVE One underpinning of this report is a systems perspective. No biometric technology, whether aimed at increasing security, improving throughput, lowering cost, improving convenience, or the like, can in and of itself achieve an application goal. Even the simplest, most automated, accurate, and isolated biometric application is embedded in a larger system. That system may involve other technologies, environmental factors, appeal policies shaped by security, business, and political considerations,. Idiosyncratic appeal mechanisms, which in turn can reinforce or vitiate the performance of any biometric system. Complex systems have numerous sources of uncertainty and variability.
Consider a fingerprint scanner embedded in a system aimed at protecting access to a laptop computer. In this comparatively simple case, the ability to achieve the fingerprint scan’s security objective depends not only on the biometric technology, but also on the robustness of the computing hardware to mechanical failures and on multiple decisions by manufacturer and employer about when and how the biometric technology can be bypassed, which all together contribute to the systems context for the biometric technology. Most biometric implementations are far more complex. Typically, the biometric component is embedded in a larger system that includes environmental and other operational factors that may affect performance of the biometric component; adjudication mechanisms, usually at multiple levels, for contested decisions; a policy context that influences parameters (for example, acceptable combinations of cost, throughput, and false match rate) under which the core biometric technology operates; and protections against direct threats to either bypass or compromise the integrity of the core or of the adjudication mechanisms. Moreover, the effectiveness of such implementations relies on a data management system that ensures the enrolled biometric is linked from the outset to the nonphysical aspects of the enrolling individual’s information (such as name and allowed privileges). The rest of this report should be read keeping in mind that biometric systems and technologies must be understood and examined within a systems context. MOTIVATIONS FOR USING BIOMETRIC SYSTEMS A primary motivation for using biometrics is to easily and repeatedly recognize an individual so as to enable an automated action based on that recognition.
The reasons for wanting to automatically recognize individuals can vary a great deal; they include reducing error rates and improving accuracy, reducing fraud and opportunities for circumvention, reducing costs, improving scalability, increasing physical safety, and improving convenience. Often some combination of these will apply. For example, almost all benefit and entitlement programs that have utilized.
Biometrics have done so to reduce costs and fraud rates, but at the same time convenience may have been improved as well. See for more on the variety of biometric applications.
Historically, personal identification numbers (PINs), passwords, names, social security numbers, and tokens (cards, keys, passports, and other physical objects) have been used to recognize an individual or to verify that a person is known to a system and may access its services or benefits. For example, access to an automatic teller machine (ATM) is generally controlled by requiring presentation of an ATM card and its corresponding PIN. Sometimes, however, recognition can lead to the denial of a benefit.
This could happen if an individual tries to make a duplicate claim for a benefit or if an individual on a watch list tries to enter a controlled environment. But reflection shows that authorizing or restricting someone because he or she knows a password or possesses a token is just a proxy for verifying that person’s presence.
A password can be shared indiscriminately or a physical token can be given away or lost. Thus, while a system can be. BOX 1.3 The Variety of Biometric Applications Biometric technology is put to use because it can link a “person” to his or her claims of recognition and authorization within a particular application.
Moreover, automating the recognition process based on biological and behavioral traits can make it more economical and efficient. Other motivations for automating the mechanisms for recognizing individuals using biometric systems vary depending on the application and the context in which the system is deployed; they include reducing error rates and improving accuracy; reducing fraud and circumvention; reducing costs; improving security and safety; improving convenience; and improving scalability and practicability. Numerous applications employ biometrics for one or more of these reasons, including border control and criminal justice (such as prisoner handling and process), regulatory compliance applications (such as monitoring who has access to certain records or other types of audits), determining who should be entitled to physical or logical access to resources, and benefits and entitlement management. The scope and scale of applications can vary a great deal—biometric systems that permit access might be used to protect resources as disparate as a nuclear power plant or a local gym. Even though at some level of abstraction the same motivation exists, the systems are likely to be very different and to merit different sorts of analysis, testing, and evaluation (see for more on how application parameters can vary). The upshot of this wide variety of reasons for using biometric systems is that much more information is needed to assess the appropriateness of a given system for a given purpose beyond the fact that it employs biometric technology.
HUMAN IDENTITY AND BIOMETRICS Essential to the above definition of biometrics is that, unlike the definition sometimes used in the biometrics technical community, it does not necessarily link biometrics to human identity, human identification, or human identity verification. Rather, it measures similarity, not identity.
Specifically, a biometric system compares encountered biological/behavioral characteristics to one or more previously recorded references. Measures found to be suitably similar are considered to have come from the same individual, allowing the individual to be recognized as someone previously known to the system. A biometric system establishes a probabilistic assessment of a match indicating that a subject at hand is the same subject from whom the reference was stored. If an individual is recognized, then previously granted authorizations can once again be granted. If we consider this record of attributes to constitute a personal “identity,” as defined in the NRC report on authentication, then biometric characteristics can be said to point to this identity record.
However, the mere fact that attributes are associated with a biometric reference provides no guarantee that the attributes are correct and apply to the individual who provided the biometric reference. Further, as there is no requirement that the identity record contain a name or other social identifier, biometric approaches can be used in anonymous applications. More concisely, such approaches can allow for anonymous identification or for verification of an anonymous identity. This has important positive implications for the use of biometrics in privacy-sensitive applications.
However, if the same biometric measure is used as a pointer to multiple identity records for the same individual across different systems, the possibility of linking these records (and hence the various social identities of the same person) raises privacy concerns. See for a note on privacy. BOX 1.4 A Note on Privacy Privacy is an important consideration in biometric systems. The report Who Goes There?
Authentication Through the Lens of Privacy, focused on the intersection of privacy and authentication systems, including biometrics. Much of that analysis remains relevant to current biometric systems, and this report does not have much to add on privacy other than exploring some of the social and cultural implications of biometric systems (see ). This reliance on an earlier report does not suggest that privacy is unimportant. Rather, the committee believes that no system can be effective without considerable attention to the social and cultural context within which it is embedded.
The 2003 NRC report just referred to and its 2002 predecessor, which examined nationwide identity systems, should be viewed as companions to this report. The Fundamental Dogma of Biometrics The finding that an encountered biometric characteristic is similar to a stored reference does not guarantee an inference of individualization—that is, that a single individual can be unerringly selected out of a group of all known individuals (or, conversely, that no such individual is known). The inference that similarity leads to individualization rests on a theory that one might call the fundamental dogma of biometrics: An individual is more similar to him- or herself over time than to any one else at any time. This is clearly false in general; many singular attributes are shared by large numbers of individuals, and many attributes change significantly over an individual’s lifetime. Further, it will never be possible to prove (or falsify) this assertion precisely as stated because “anyone else” will include all persons known or unknown, and we cannot possibly prove the assertion for those who are unknown. In practice, however, we can relate similarity to individualization in situations where.
An individual is more likely similar to him- or herself over time than to anyone else likely to be encountered. This condition, if met, allows us to individualize through similarity, but with only a limited degree of confidence, based on knowledge of probabilities of encounters with particular biometric attributes. The goal in the development and applications of biometric systems is to find characteristics that are stable and distinctive given the likelihood of encounters. If they can be found, then the above conditions are satisfied and we have a chance of making biometrics work—to an acceptable degree of certainty—to achieve individualization.
A better fundamental understanding of the distinctiveness of human individuals would help in converting the fundamental dogma of biometrics into grounded scientific principles. Such an understanding would incorporate learning from biometric technologies and systems, population statistics, forensic science, statistical techniques, systems analysis, algorithm development, process metrics, and a variety of methodological approaches.
However, the distinctiveness of biometric characteristics used in biometric systems is not well understood at scales approaching the entire human population, which hampers predicting the behavior of very large scale biometric systems. The development of a science of human individual distinctiveness is essential to the effective and appropriate use of biometrics as a means of human recognition and encompasses a range of fields. This report focuses on the biometric technologies themselves and on the behavioral and biological phenomena on which they are based.
These phenomena have fundamental statistical properties, distinctiveness, and varying stabilities under natural physiological conditions and environmental challenges, many aspects of which are not well understood. 7 There have been several comprehensive examinations of biometrics technologies and systems over the years. See, for example, J.L. Maltoni, and D. Maio, eds., Biometric Systems: Technology, Design, and Performance Evaluation, London: Springer (2005); J. Woodward, Jr., N.
Orlans, and P. Higgins, Biometrics: Identity Assurance in the Information Age, New York: McGraw-Hill/Osborne Media (2002); and A.K.
Bolle, and S. Pankanti, eds., Biometrics: Personal Identification in a Networked Society, Norwell, Mass.: Kluwer Academic Press (1999). The National Science and Technology Council also recently. Sample Operational Process The operational process typical for a biometric system is given in. The main components of the system for the purposes of this discussion are the capture (whereby the sensor collects biometric data from the subject to be recognized), the reference database (where previously enrolled subjects’ biometric data are held), the matcher (which compares presented data to reference data in order to make a recognition decision), and the action (whereby the system recognition decision is revealed and actions are undertaken based on that decision. This diagram presents a very simplified view of the overall system. The operational efficacy of a biometric system depends not only on its technical components—the biometric sample capture devices (sensors) and the mathematical algorithms that create and compare references—but also on the end-to-end application design, the environment in which the biometric sensor operates, and any conditions that impact the behavior of the data subjects, that is, persons with the potential to be sensed.
For example, the configuration of the database used to store references against which presented data will be compared affects system performance. At a coarse level, whether the database is networked or local is a primary factor in performance. Networked databases need secure communication, availability, and remote access privileges, and they also raise more privacy challenges than do local databases.
Local databases, by contrast, may mean replicating the reference database multiple times, raising security, consistency, and scalability challenges. In both cases, the accuracy and currency of any identification data associated with reference. 8 The data capture portion of the process has the most impact on accuracy and throughput and has perhaps been the least researched portion of the system.
The capture process, which involves human actions (even in covert applications) in the presence of a sensor, is not well understood. While we may understand sensor characteristics quite well, the interaction of the subject with the sensors merits further attention.
See for more on research opportunities in biometrics. 9 Both the NRC report Who Goes There? (2003) and the EC Data Protection Working Party discuss the implications of centralized or networked data repositories versus local storage of data.
The latter is available. Measures of Operational Efficacy Key aspects of operational efficacy include recognition error rates; speed; cost of acquisition, operation, and maintenance; data security and privacy; usability; and user acceptance. Generally, trade-offs must be made across all of these measures to achieve the best-performing system consistent with operational and budgetary needs. For example, recognition error rates might be improved by using a better but more time-consuming enrollment process; however, the time added to the enrollment process could result in queues (with loss of user acceptance) and unacceptable costs. In this report the committee usually discusses recognition error rates in terms of the false match rate (FMR; the probability that the matcher recognizes an individual as a different enrolled subject) and the false nonmatch rate (FNMR; the probability that the matcher does not recognize a previously enrolled subject). FMR and FNMR refer to errors in the matching process and are closely related to the more frequently reported false acceptance rate (FAR) and the false rejection rate (FRR).
FAR and FRR refer to results at a broader system level and include failures arising from additional factors, such as the inability to acquire a sample. The committee uses these terms less frequently as they can sometimes intro. Duce confusion between the semantics of “acceptance” and “rejection” in terms of the claimed performance for biometric recognition versus that for the overall application. For example, in a positive recognition system, a false acceptance occurs when subjects are recognized who should not be recognized—either because they are not enrolled in the system or they are someone other than the subject being claimed. In this case the sense of false acceptance is aligned for both the biometric matching operation and the application function. In a system designed to detect and prevent multiple enrollments of a single person, sometimes referred to as a negative recognition system, a false acceptance results when the system fails to match the submitted biometric sample to a reference already in the database.
If the system falsely matches a submitted biometric sample to a reference from a different person, the false match results in a denial of access to system resources (a false rejection). Variability and Uncertainty Variability in the biometric data submitted for comparison to the enrolled reference data can affect performance. As mentioned above, the matching algorithm plays a role in how this variability is handled.
However, many other factors can influence performance, depending on how the specific biometric system is implemented. How To Decode Php Files That Encoded By Zend Encoder. Generally speaking, biometric applications automatically capture aspects of one or more human traits to produce a signal from which an individual can be recognized. This signal cannot be assumed to be a completely accurate representation of the underlying biometric characteristic.
Biometric systems designers and experts have accepted for some time that noise in the signal occurs haphazardly; while it can never be fully controlled, it can be modeled probabilistically. Also, not all uncertainty about biometric systems is due to random noise. Uncertainty pervades a biometric system in a number of ways. Several potential sources of uncertainty or variation are discussed here and should be kept in mind when reading the rest of this report. These sources are listed based on the order of events that take place as an individual works with the system to gain recognition.
• Depending on the biometric modality, information content presented by the same subject at different encounters may be affected by changes in age, environment, stress, occupational factors, training and prompting, intentional alterations, sociocultural aspects of the situation in which the presentation occurs, changes in human interface with the bio. Metric system, and so on.
These factors are important both at the enrollment phase and during regular operation. The next section describes within-person and between-person variability in depth. • Sensor operation is another source of variability. Sensor age and calibration can be factors, as well as how precisely the system-human interface at any given time stabilizes extraneous factors. Sensitivity of sensor performance to variation in the ambient environment (such as light levels) can play a role. • The above sources may be expected to induce greater variation in the information captured by different biometric acquisition systems than in the information captured by the same system.
Other factors held constant, information on a single subject captured from repeated encounters with the same sensor will vary less than that captured from different sensors in the same system, which will vary less than that captured from encounters with different systems. In addition to information capture, performance variation across biometric systems and when interfacing components of different systems depends on how the information is used, including the following: • Differences in feature extraction algorithms affect performance, with effects sometimes aggravated by the need for proprietary systems to be interoperable. • Differences between matching algorithms and comparison scoring mechanisms.
How these algorithms and mechanisms interact with the preceding sources of variability of information acquired and features extracted also contributes to variation in performance of different systems. For instance, matching algorithms may differ in their sensitivity to biological and behavioral instability of the biometric characteristic over time, as well as the characteristic’s susceptibility to intentional modification. • The potential for individuals attempting to thwart recognition for one reason or another is another source of uncertainty that systems should be robust against. See below for a more detailed discussion on security for biometric systems.
In light of all of this, determining an appropriate action to take—where possible actions include to recognize, to not recognize, or to transition the system to a secondary recognition mechanism based on the signal from a biometric device—involves decision making under uncertainty. Within-Person Variation Ideally, every time we measure the biometric trait of an individual, we should observe the same patterns. In practice, however, the different samples produce different patterns, which result in different digital representations (references).
Such within-person variation, sometimes referred to as “intraclass variation,” typically occurs when an individual interacts differently with the sensor (for example, by smiling) or when the biometric details of a person (for example, hand shape and geometry or iris pigmentation details) change over time. The sensing environment (for example, ambient lighting for a face and background noise for a voice) can also introduce within-person variation.
There are a number of ways to reduce or accommodate such variation, including controlled acquisition of the data, storage of many references for every user, and systematic updating of references. Reference updating, although essential to any biometric system since it can help account for changes in characteristics over time, introduces system vulnerabilities. Some biometric traits are more likely to change over time than others. Face, hand, and voice characteristics, in particular, can benefit from suitably implemented reference update mechanisms.
Within-person variation can also be caused by behavioral changes over time. Between-Person Variation Between-person variation, sometimes referred to as “interclass variation,” refers generally to person-to-person variability. Since there is an inherent similarity between biometric traits among some individuals (faces of identical twins offer the most striking example), between-person variation between two individuals may be quite small. Also, a chosen digital representation (the features) for a particular biometric trait may not very effective in separating the observed patterns of particular subjects. In contrast, demographic heterogeneity among enrolled subjects in a biometric system database may contribute to large between-person variation in measurements of a particular biometric trait, although fluctuations in the sensing environment from which their presentation samples are obtained may contribute to large within-person variation as well.
It is the magnitude of within-person variation relative to between-person variation (observed in the context of a finite range of expression of human biometric traits) that determines the overlap between distributions of biometric measurements from different individuals and hence limits. Crack Topsolid 2011 Gratuities here. The number of individuals that can be discriminated and recognized by a biometric system with acceptable accuracy.
When within-person variation is small relative to between-person variation, large biometric systems with high accuracy are feasible because the distributions of observed biometric data from different individuals are likely to remain widely separated, even for large groups. When within-person variation is high relative to between-person variation, however, the distributions are more likely to impinge on each other, limiting the capacity of a recognition system. In other words, the number of enrolled subjects cannot be arbitrarily increased for a fixed set of features and matching algorithms without degrading accuracy. When considering the anticipated scale of a biometric system, the relative magnitudes of both within-person and between-person variations should be kept in mind. Stability and Distinctiveness at Global Scale Questions of variation and uncertainty become challenging at scale. In particular, no biometric characteristic, including DNA, is known to be capable of reliably correct individualization over the size of the world’s population. Factors that make unlikely the discovery of a characteristic suitably stable and distinctive over such a large population include the following: • Individuals without traits.
Almost any trait that can be noninvasively observed will fail to be exhibited by some members of the human population, because they are missing the body part that carries the trait, because environmental or occupational exposure has eradicated or degraded the trait, or because their individual expression of the trait is anomalous in a way that confuses biometric systems. • Similar individuals. In sufficiently small populations it is highly likely that almost any chosen trait will be sufficiently distinctive to distinguish individuals. As populations get larger, most traits (and especially most traits that can be noninvasively observed) may have too few variants to guarantee that different individuals are distinguishable from one another. The population statistics for most biometric traits are poorly understood.
• Feature extraction effects. Even in cases where a biometric trait is distinctive, the process of converting the analog physical property of a human to a digital representation that can be compared against the properties of other individuals involves loss of detail—that is, information loss—and introduction of noise, both of which can obscure distinctions between individuals. However, the lack of an entirely stable and distinctive characteristic at scale need not stand in the way of effective use of biometrics if the system is well designed. Some biometric systems might not have to deal with all people at all times but might need only to deal with smaller groups of people over shorter periods of time.
It may be possible to find traits that are sufficiently stable and distinctive to make many types of applications practicable. Implicit in all biometric systems are estimated probabilities of the sameness and difference of source of samples and stored references, separately for presenters of different types, such as residents and impostors. This leads to the explicit use of ratios of probabilities in some biometric recognition algorithms.
Because assessing the likelihood that a sample came from any particular reference may involve computing similarity to many references through use of ratios of probabilities or other normalization techniques, it cannot be strictly said that any form of recognition involves comparison of only one sample to one known reference. However, verification of a claim of similarity of a sample with a specific reference may appear to those unfamiliar with the algorithmic options to involve only a single comparison. This form of verification is often referred to as “one to one.” Verification of claims of similarity to an unspecific reference is often referred to as a “one-to-many” application because many comparisons to assess similarity over the enrolled individuals are required. 11 A biometric modality is the combination of a biometric trait, sensor type, and algorithms for extracting and processing the digital representations of the trait. When any two of these three constituents differ from one system to the next, the systems are said to have different modalities. For example, infrared facial recognition and iris recognition are different modalities since the trait and the algorithms differ even if the same camera is used.
12 DNA could be considered a biometric modality if the technologies for it can be sufficiently automated. However, this report focuses on those modalities and systems for which automated technologies are further along in development and deployment. Jain, Arun Ross, and Salil Prabhakar, An introduction to biometric recognition, IEEE Transactions on Circuits and Systems for Video Technology, Special Issue on Image- and Video-Based Biometrics 14(1) (2004). Face Static or video images of a face can be used to facilitate recognition. Modern approaches are only indirectly based on the location, shape, and spatial relationships of facial landmarks such as eyes, nose, lips, and chin, and so on. Signal processing techniques based on localized filter responses on the image have largely replaced earlier techniques based on representing the face as a weighted combination of a set of canonical faces.
Recognition can be quite good if canonical poses and simple backgrounds are employed, but changes in illumination and angle create challenges. The time that elapses between enrollment in a system and when recognition is attempted can also be a challenge, because facial appearance changes over time. Fingerprints Fingerprints—the patterns of ridges and valleys on the “friction ridge” surfaces of fingers—have been used in forensic applications for over a century. Friction ridges are formed in utero during fetal development, and even identical twins do not have the same fingerprints.
The recognition performance of currently available fingerprint-based recognition systems using prints from multiple fingers is quite good. One factor in recognition accuracy is whether a single print is used or whether multiple or tenprints (one from each finger) are used. Multiple prints provide additional information that can be valuable in very large scale systems. Challenges include the fact that large-scale fingerprint recognition systems are computationally intensive, particularly when trying to find a match among millions of references. Hand Geometry Hand geometry refers to the shape of the human hand, size of the palm, and the lengths and widths of the fingers. Advantages to this modality are that it is comparatively simple and easy to use. However, because it is not clear how distinctive hand geometry is in large populations, such systems are typically used for verification rather than identification.
Moreover, because the capture devices need to be at least the size of a hand, they are too large for devices like laptop computers. Comparison of Modalities Each biometric modality has its pros and cons, some of which were mentioned in the descriptions above.
Moreover, even if some of the downsides could be overcome, a modality itself might have inherent deficiencies, although very little research into this has been done. Therefore, the choice of a biometric trait for a particular application depends on issues besides the matching performance. Raphael and Young identified a number of factors that make a physical or a behavioral trait suitable for a biometric application. The following seven factors are taken from an article by Jain et al.: • Universality.
Every individual accessing the application should possess the trait. • Uniqueness. The given trait should be sufficiently different across members of the population. • Permanence. The biometric trait of an individual should be sufficiently invariant over time with respect to a given matching algorithm.
A trait that changes significantly is not a useful biometric. • Measurability. It should be possible to acquire and digitize the biometric trait using suitable devices that do not unduly inconvenience the.